Comparing Structure and Conformation of Diphtheria Toxin With Its Non-toxic Mutant (E349K) at 300 K Using Molecular Dynamics Simulations
Avicenna J Clin Microbiol Infect, 6(4), 111-117; DOI:10.34172/ajcmi.2019.20
Original Article
Comparing Structure and Conformation of Diphtheria Toxin With Its Non-toxic Mutant (E349K) at 300 K Using Molecular Dynamics Simulations
Soheila Ghaderi1, Mohammad Reza Bozorgmehr2, Shirin Tarahomjoo3*, Abdolrez Movahedi1, Majid Esmaelizad1, Mojtaba Noofeili4
1
Division of Central Laboratory, Department of Biotechnology and Central Laboratory, Razi Vaccine and Serum Research Institute, Agricultural Research, Education and Extension Organization (AREEO), Karaj, Iran
2
Department of Chemistry, Faculty of Science, Mashhad Branch, Islamic Azad University, Mashhad, Iran
3
Division of Genomics and Genetic Engineering, Department of Biotechnology and Central Laboratory, Razi Vaccine and Serum Research Institute, Agricultural Research, Education and Extension Organization (AREEO), Karaj, Iran
4
Department of DTP Vaccine Production, Razi Vaccine and Serum Research Institute, Agricultural Research, Education and Extension Organization (AREEO), Karaj, Iran
*Corresponding author:
Shirin Tarahomjoo Division
of Genomics and Genetic
Engineering, Department of
Biotechnology, Razi Vaccine
and Serum Research Institute,
Agricultural Research,
Education and Extension
Organization (AREEO), Karaj,
Iran,
Tel: 98-263-4570038,
Fax: 98-263-4552194, Email: starahomjoo@gmail.com
Abstract
Background: The molecular dynamics simulations have provided detailed information on the fluctuations and conformational changes of proteins. Mutation of GLu349 of diphtheria toxin (DT) to Lys inhibits the molecular cytotoxicity in mammalian cells. In this work, we thus aimed to evaluate the effects of the mutation on the structure and the conformation of DT using molecular dynamic simulations.
Methods: PYMOL system was used for introducing the mutant amino acid to DT. Three dimensional structures were visualized using YASARA. The protonation fixing process was done using H++ server. SPD viewer was then applied to retrieve missing hydrogen atoms. Molecular dynamic simulations were also carried out using GROMACS software. Finally, RMSF, Rg, and RMSD graphs were drawn by Sigma Plot using GROMACS outputs.
Results: The results of our analysis indicated that amino acid residue fluctuations in the catalytic domain (C) of DT were greater than those of its mutant (E349K). Moreover, residue fluctuations in the region, including Ile344- val347, Tyr514-Ser525, and Ile533-Lys534 of DT were greater than those of the mutant (E349K). However, residue fluctuations in the region including Cys186-Cys201 and Glu349-Val351 of DT were lower than those of E349K. In addition, the disulfide bridge (Cys186–Cys201) was formed in DT, whereas it was not observed in the mutant. The secondary structure analysis showed that the beta-sheet content of E349K decreased compared with DT. Additionally, the conformation of DT was different from that of E349K in the hinge loop regions (Ala379–Thr386 and Tyr514-Ser525). The radius of gyration (Rg) and the root mean square deviation (RMSD) of E349K were greater than those of DT. The conformational stability and compactness of DT were higher than those of E349K.
Conclusions: The method used in this study can demonstrate the structural details of toxins as important aspects to predict activities of the receptor binding and the catalytic domains. Therefore, it can be used to evaluate conformational changes of toxins as well as other vaccine candidates.
Keywords: Theoretical biology, Secondary structure, Targeted mutagens
Background
Diphtheria is an infectious disease caused by strains of Corynebacterium diphtheriae. This phage carries tox gene, which encodes diphtheria toxin (DT) (1,2). The transcription of this gene is activated by a lack of iron. The toxin is responsible for major symptoms, morbidity and mortality of the disease. The infection spreads over the pharynx, larynx and tonsils. The treatment of diphtheria is based on subcutaneous injections of immunoglobulins purified from the serum of horses immunized against DT (3). In 1923, Ramon described the preparation of toxoid as a formaldehyde inactivated form of DT, which is still the basis for today’s vaccine against diphtheria (4). DT was purified and characterized as a protein in the 1930s by Eaton (5) and Pappenheimer (6). The tox gene encoding DT was sequenced in 1983 (7,8). Then, Choe et al published an article entitled “The Crystal Structure of DT” in 1992, which enabled them to propose a detailed model of the molecular mechanism of action of DT at an atomic resolution (9). It became possible to test and refine this model by site-directed mutagenesis and protein engineering (10).
DT is a protein of 535 amino acids organized in three structural domains of equivalent sizes, ranging from the N- to the C-terminus: 1- the catalytic (C) (1-188), The C domain contains 7 short alpha-helices surrounding two beta-sheets of 5 and 3 strands. The disulfide bridge Cys186–Cys201 connects C-terminus of the C domain to N-terminus of the T domain. 2- the transmembrane or translocation (T), T is organized as a bundle of 10 alpha-helices named TH1 to TH9 and TH5’ (between TH5 and TH6). 3- the receptor-binding (R) domains, R has a flattened beta roll topology containing 11 strands that roughly resembles the fold of the immunoglobulin variable domain. Proteolysis allows the C domain to dissociate from the T and R domains once the disulfide bridge between residues 186 and 201 is reduced while disulfide bonds are introduced inside the C domain block translocation (11). This disulfide bond does not cross the membrane before the rest of the domain (12-14). It is then reduced on the cytoplasmic side of the membrane, at an early stage of the process of translocation, before the C domain is fully translocated (11). The second disulfide bridge of DT, connecting Cys461 to Cys471, is located within the R domain. Therefore, C corresponds to fragment A, and T and R to fragment B according to the classical denomination used for bacterial toxins with intracellular targets (15). Interestingly, the binding of DT to heparin-binding epidermal growth factor (HB-EGF) is accompanied by a large structural change in the toxin (16). The R domain swivels 180° around the natural hinge formed by residues 379–386 linking T to R, just as the page of a book opens. As a consequence, the R domain is located far from the C and T domains (17). Based on the refined DT structures and hydrophobicity, the analysis proposed the four potential transmembrane helices to be represented as cylinders and labeled A (residues 269- 289), B (residues 301-321), C (residues 328-348) and D (residues 351-371). The open monomer exposes a polar surface on helices B and D, indicated as striped patches. Acidic residues (Asp 290, Glu292, Asp 295, Glu298, Glu349, and Asp 352) in the helices A and B and helices C and D are represented as protonated carboxyl groups (15). The mutation of Glu349 to Lys in the C-D loop impairs the DT cytotoxicity by reducing low pH-triggered translocation across membranes supporting the hypothesis that protonation of acidic residues in the T domain is involved (18). Attempts to produce synthetic or recombinant vaccines from fragments of the DT have been described. The synthetic peptide (residues 188–201 of DT) corresponding to the loop connecting the C domain to the T domain elicited protection against DT in guinea pigs (19,20). The past 15 years have seen the development of many new applications for DT. When isolated from each other, the three domains of DT maintain their structure and function. These domains were isolated or combined in pair and were associated with other proteins or compounds which are now exploited for their biological properties, leading to new biotechnological tools and new therapeutics. One of these applications has led to an FDA-approved therapy for lymphomas (21). Based on the results of previous empirical experiments, the E349K mutation alone inhibited cytotoxicity and membrane translocation in mammalian cells and lethality of Escherichia coli but did not affect enzymatic activity or receptor binding. Polar helices may then span the bilayer with the TL5 tip exposed to the aqueous phase on the opposite face of the membrane. The E349K mutation, by introducing a positively charged group, would be expected to impede the insertion of the TL5 loop and inhibit insertion-dependent functions, including cytotoxicity (18). Genetically modified toxoids and their fragments are currently regarded as more promising components for vaccines than the chemically modified analogues of the natural toxins since they are cheaper, quite immunogenic, and cause fewer complications in multiple vaccinations (22).
Using Bioinformatics methods and molecular dynamics simulations (MD), the targeted mutagens are important tools in the theoretical study of biological molecules. This computational method calculates the time-dependent behavior of a molecular system. MD simulations have provided detailed information on the fluctuations and conformational changes of proteins and nucleic acids. These methods are now routinely used to investigate the structure, dynamics and thermodynamics (23). Extended MD simulations were liable to play a vital role in modeling the long-timescale native and mutant state dynamics and their association with protein function (24).
In this study, we demonstrated conformational changes of DT and its mutant (E349K) using the molecular dynamics simulations. Moreover, we described the molecular structures of the proteins, thereby explaining precisely how the structural change of DT through mutation influenced the toxin function. The mutation in fragment B of DT in E349K resulted in nontoxicity. In addition, E349K did not show immunogenicity. Comparing the structures of DT and E349K can thus result in the design of non-toxic DT mutants with appropriate vaccination efficiency. This method can be used for the identification and recognition of the amino acid residue fluctuations of the bacterial toxins and the prediction of the activities of the catalytic and receptor binding domains.
Materials and Methods
Preparing Input Structures
The DT structure was retrieved from Protein Data Bank (PDB). The protein with a PDB ID of 1F0L was selected (15). The introduction of the mutant amino acid to DT (E349K) was carried out using PYMOL and the obtained three-dimensional structure of mutant was compared with the DT structure using YASARA software. Molecular graphics program PYMOL and YASARA are programs widely used for viewing 3D structures of proteins and the related analysis e.g., amino acid sequence alignments, analysis of elements forming secondary and tertiary structures of proteins, and comparisons of protein structures. The protonation fixing process was done with DT and the mutant protein (E349K) to prepare the structures in the neutral pH (6.5) as the input for molecular dynamics simulation using H++ server(25). This online server adjusts the protein structure in the desired pH based on the pKa and H+ concentration. The resulted structure should be viewed by SPD viewer to retrieve missing hydrogen atoms and thus making the atomic dimensions appropriate to apply in molecular dynamics simulations.
Initiating Molecular Dynamics Simulations
GROMACS is a free software for optimizing structures of macromolecules based on their thermodynamic properties such as molecular internal energy. For this purpose, the atomic dimension obtained as described above is subjected to GROMACS and is improved iteratively through minimizing the molecular internal energy based on a defined force field. Force fields are composed of different types of atomic energies and relied on considering experimental estimates. The molecular dynamics simulations were done by GROMACS 4.5.4 package using AMBER99SB force field, periodic boundary conditions, and two simulation boxes with dimensions of 9.5 × 9.5 × 9.5 nm (25). The periodic boundary conditions are used to prevent the problems arisen from the boundary effects of simulation boxes. Then, the boxes were filled with an appropriate number of water molecules. In order to neutralize the system, the appropriate numbers of Na and Cl ions were added to each box. To eliminate any undesirable contacts between atoms and initial kinetic energy in the simulation boxes, the energy was minimized by applying the steepest descent algorithm.
Equilibrating and Running Molecular Dynamics Simulations
Two systems defined above for DT and the mutant protein were equilibrated in two stages in GROMACS under 5 ns as the required time, NVT and NPT simulations with temperature and pressure fixed at 300 K and 1 bar, respectively. The pressure was controlled at 1 bar and the temperature was retained at 300 K using Parrinello- Rahman Barostat (26) and V-rescale thermostat, respectively (27). NVT is used in GROMACS to keep particle numbers, volume, and temperature constant. Furthermore, NPT results were used to keep particle numbers, pressure, and temperature constant. For each component of the systems, PME algorithm (28) was applied to estimate the electrostatic interactions. LINCS algorithm (29) was employed to fix the chemical bonds in the atoms of the protein and the SETTLE algorithm was used to fix the solvent molecules (30). The simulations were run for 20 ns, and a time step of 2 fs was used throughout the simulations. All simulations were repeated to test the convergence of the results. Sigma Plot was used to create the root mean square fluctuation (RMSF), radius of gyration (Rg), and root mean square deviation (RMSD) graphs using GROMACS outputs.
Results
RMSF Data
After performing the molecular dynamics simulations in this work, we tried to find fluctuations of the key residues in DT and E349K. RMSF was measured, which indicated deviations of particle positions from some reference positions and was used as a criterion for the residue flexibility.
Six regions showed considerable fluctuations in DT and E349K (Figure 1A). Fluctuations of amino acid residues in the first region (C domain) spanning Gly1-Cys186 of DT were greater than those of E349K. In the second region (Cys186-Cys201), the Cys186 fluctuation of DT (RMSF = 0.1570 nm) was considerably lower than that of E349K (RMSF = 0.1873 nm). In the third region including Ile344-val347, the fluctuation of residue Ile344 of DT (RMSF = 0.1178 nm) was greater than that of E349K (RMSF = 0.0940 nm). In the fourth region including Glu349-Val351, fluctuations of residues Glu349 and Leu350 of DT were lower than those of E349K. Glu- 349 and the other acidic residue (Asp-352) were within the helical transmembrane domain that resided on the opposite side of the C and R domains in the membrane.
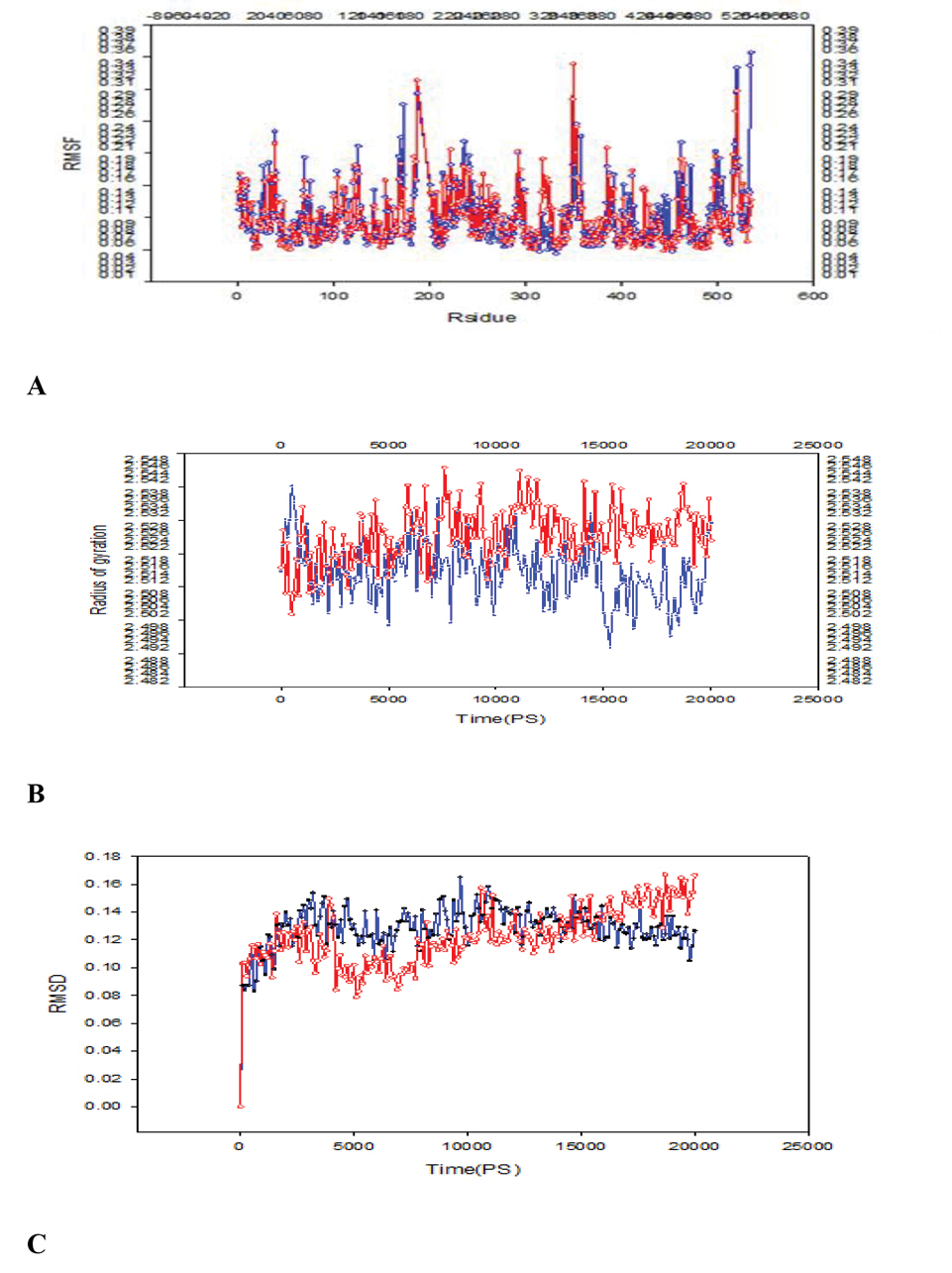
A: Comparison of RMSF of E349K and DT residues; B:Comparison of Rg of E349K and DT residues; C:Comparison of RMSD of E349K and DT residues; the solid line and the dotted line showed the mutant and DT, respectively.
In the fifth region (Tyr514-Ser525), the fluctuation of His520 of DT (RMSF = 0.3339 nm) was greater than that of E349K (RMSF = 0.1636 nm).
The sixth region consisted of Ile533 and Lys534 in which Ile-533 was the nonpolar amino acid and Lys-534 was the charged residue. In this region as a part of the R domain, the fluctuations of residues of DT were greater than those of E349K. The RMSF values showed that residue fluctuations in the C domain and R domain of DT were greater than those of E349K.
Radius of Gyration Data
Rg is the mass weighted root mean square distance of a collection of atoms from their common center of mass. It is a measure of the compactness of the system, which is correlated to the protein stability (31,32). The plot of Rg at 20 ns as the simulation time was given in Figure 1B. Rg of the mutant DT increased during the simulation and thus the protein compactness and the stability decreased.
RMSD Data
RMSD refers to the root mean square deviation (33). The plots of RMSD were obtained from molecular dynamics as shown in (Figure 1C). The overall RMSD was suitable and a minimum deviation was observed for the DT in comparison to the mutant (E349K). DT and E349K showed divergence from starting states, resulting in the final RMSD of 0.12 nm and 0.17 nm, respectively.
Secondary Structures
The secondary structure of DT and E349K were evaluated by Kabsch–Sander method (34) and Polyview server (24). These elements were demonstrated in Figure 2. Percentages of the elements for DT and E349K were reported in Table 1. The secondary structure of DT was composed of β-sheets (29.0%) and α-helix (28.8%). On the other hand, the α-helix content of the mutant increased l.2% and its β-sheet content decreased 1.7%. The percentage of turn in E349K decreased compared with that in DT. The change in the β-sheet content affects protein stability.
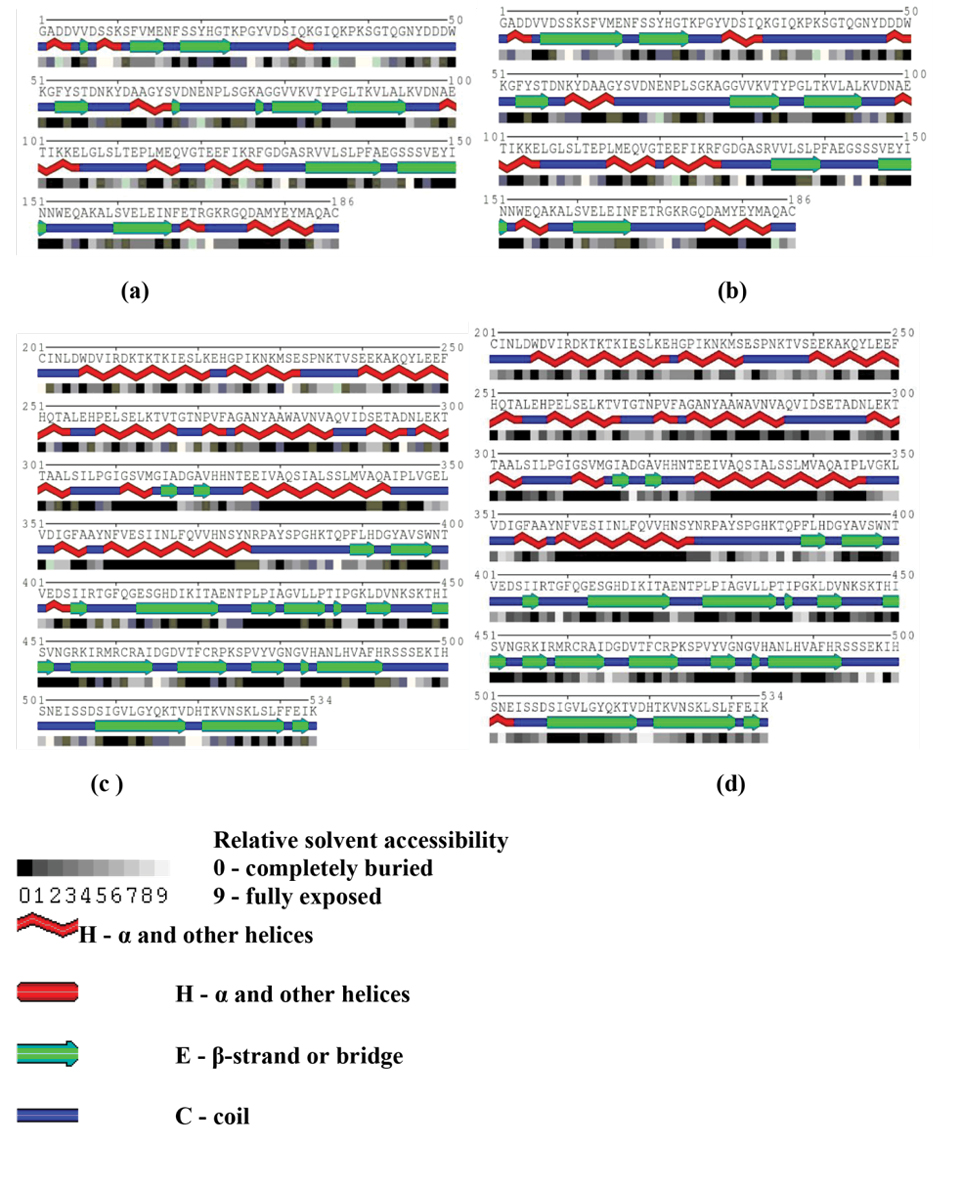
A: The secondary structure of chain A of DT in simulated systems; B:The secondary structure of chain A of E349K in simulated systems; C:The secondary structure of chain B of DT in simulated systems; D:The secondary structure of chain B of E349K in simulated systems.
Table 1. Comparison of Secondary Structure of the Diphtheria Toxin Types, Mutant (E349K) and Wild, after 20 ns Timescale Simulation in Water and at 300 K.
|
Secondary structure
|
DT
|
Mutant (E349K)
|
Helix |
28.8% |
30.6% |
Sheet |
29.9% |
27.3% |
Turn |
12.3% |
8.1% |
Coil |
26.6% |
31.3% |
Tertiary Structures
The disulfide bridge Cys186–Cys201 connecting the C-terminus of the C domain to N-terminus of the T domain was not present in E349K. This disulfide bridge is essential for the DT activity. However, it was observed in DT. In addition, conformational changes of the E349K structure were observed in the R domain consisting of the β hairpin and hinge loops (residues 379-386) (Figure 3).
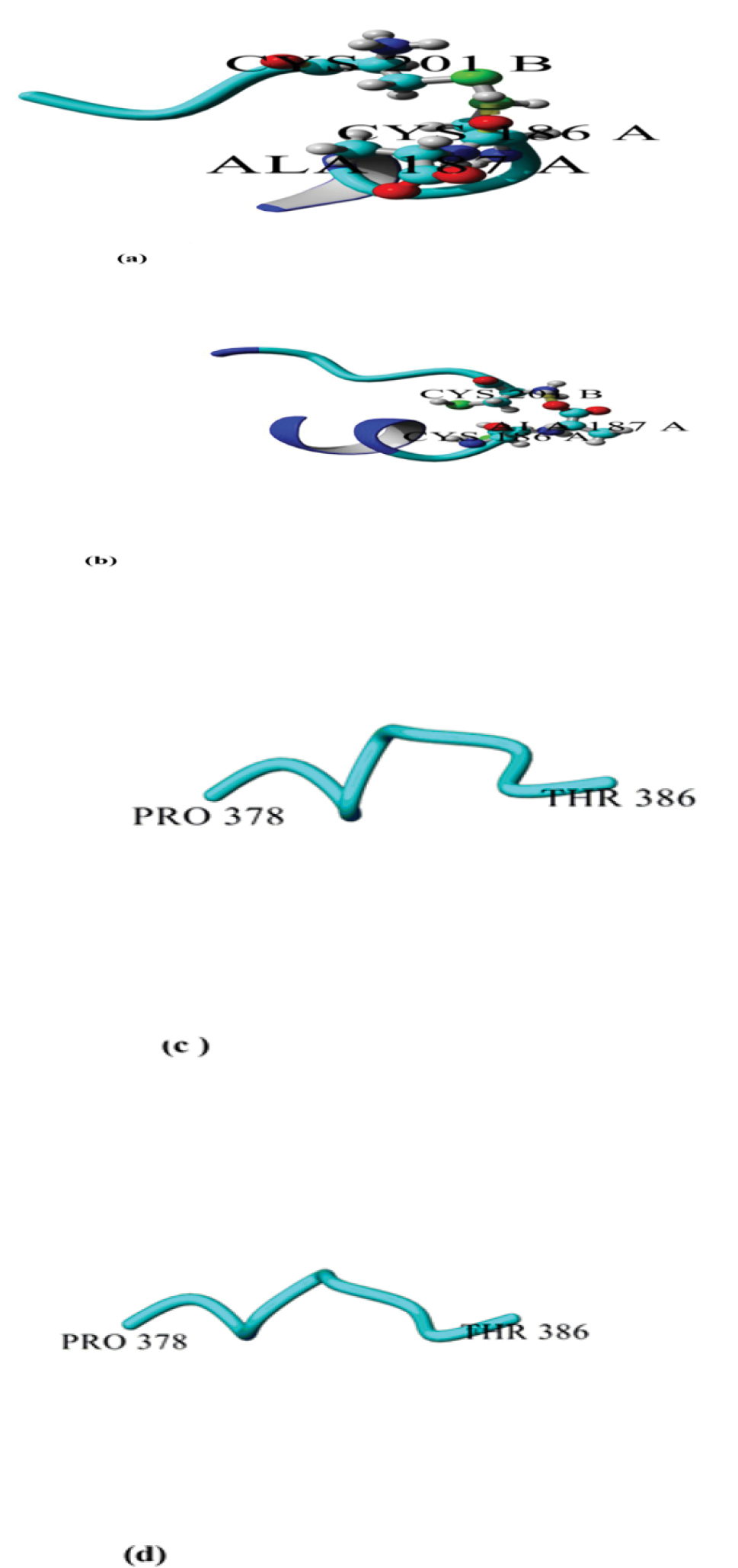
Snapshots of DT and E349K after 20 ns simulation at 300 K; A:disulfide bridge Cys186–Cys201 linked C to T domain of DT; B:disulfide bridge Cys186–Cys201 linked C to T domain of the mutant (E349K). C:hinge loop between the T and R domains (residues 378- 386) of DT; D:hinge loop between the T and R domains (residues 378-386) of the mutant (E349K).
In order to compare tertiary structures of DT and E349K, their contact map was evaluated using CM View (version 1.1.1) (35) as shown in Figure 4. The contact map of chain B of DT and the mutant showed that there were remarkable differences in the region Gly348-Lys522 consisting of the coil and the β-sheet structure. The most important difference between structures was observed in residues 348-349 of the T domain and in the R domain residue 522.
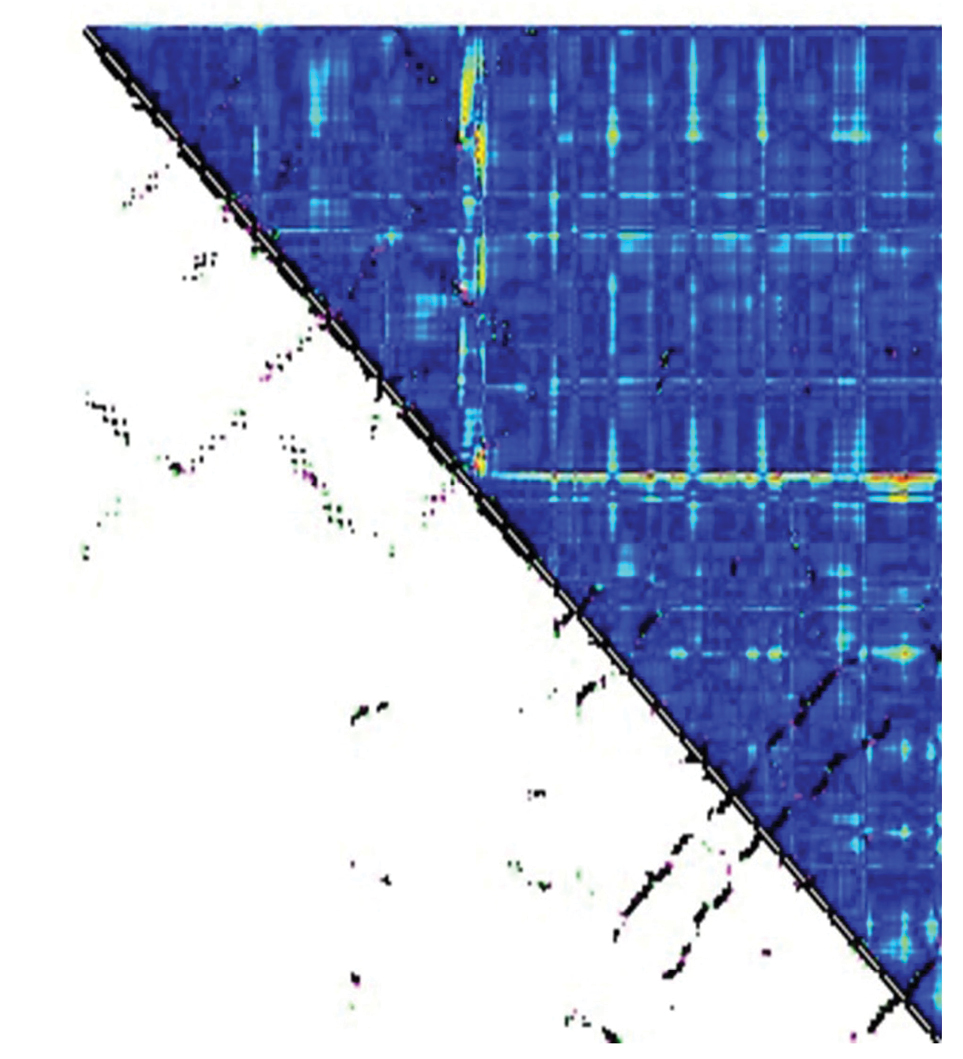
Comparison of contact map for chain B of DT and E349K; Black points showed the common contacts; pink dots showed the contacts in DT, which did not exist in the mutant. Green dots showed the contacts in the mutant, which did not exist in DT. The red and blue color intensities showed the differences between structures. Blue sections demonstrated the the unchanged contacts between two structures, and red sections showed the contacts with the most difference between the two structures.
Discussion
RMSF is an indicator of the macromolecule flexibility. High flexible domains show high fluctuations and thus their structures are in unfolded states. The highly fluctuating residues in the sequence are unstable and are probably the initial points of the protein denaturation (36,37). In this work, we have found that RMSF fluctuations of residues in C and R domains of DT including Gly1-Cys186 and Lys534-Ser535 were greater than those of E349K. On the other hand, fluctuations of Glu349-Val351 from E349K were greater than those of DT. These fluctuations were present in the T domain; therefore, they did not affect the R domain and sequential binding of substrates.
Based on the results of molecular dynamics simulation, increasing Rg resulted in decreasing the compactness of E349K compared with DT. These results can be used to reveal differences between structures of DT and its mutant as well as their functional aspects. Furthermore, the results predicted the lack of the receptor binding and the catalytic activities of E349K.
Larger Rg values show that the structure is less compact (38). The compactness has been defined as the ratio of accessible surface area of a protein to the surface area of the ideal sphere of the same volume (39,40). The molecular spatial packing of amino acid residues is an important aspect of protein stability. A compact packing of amino acid residues is known to influence both the stability and the folding rate of proteins. Rg is a parameter that describes the equilibrium conformation of a total system. Hence, it provided an observation into global dimension of protein (41). Our results indicated that the Rg of E349K was greater than that of DT and therefore its compactness was lower than that of DT. As a result, the stability of DT was higher than that of E349K. Moreover, the observed decrease in the β-sheet content of the mutant (E349K) compared with DT can correlate with the stability reduction of the protein.
The disulfide bridge Cys186–Cys201 of DT was absent from the mutant. The missing disulfide bridge can negatively affect the biological activity of E349K. Reduction of this disulfide bond results in the separation of the C domain from T and R domains (corresponding to the so-called fragment A and fragment B of bacterial toxins with intracellular targets) and a loss of toxicity (17). The conformation difference in the hinge loop Pro378–Thr386 was visible between E349K and DT. These results confirmed that changing conformation in DT allows the interaction of the T domain with the cell membrane while the R domain remains bound to pro- HB-EGF when the toxin reaches the acidic pH of the endosome (16).
Conclusions
The conformational stability and compactness of DT were higher than those of E349K. The method used in this study can, therefore, be used to evaluate conformational changes of toxins and proteins as well as vaccine candidates. It demonstrated the structural detail of toxins as an important aspect to predict activities of the receptor binding and the catalytic domains.
Conflict of Interest Disclosures
The authors declare no conflict of interests related to this work.
Ethical Approval
Not applicable.
References
- Gill DM, Uchida T, Singer RA. Expression of diphtheria toxin genes carried by integrated and nonintegrated phage beta. Virology 1972;50(3):664-8. doi: 10.1016/0042- 6822(72)90420-5. [Crossref]
- Holmes RK. Biology and molecular epidemiology of diphtheria toxin and the tox gene. J Infect Dis 2000;181 Suppl 1:S156- 67. doi: 10.1086/315554. [Crossref]
- Murphy JR, Michel JL, Teng M. Evidence that the regulation of diphtheria toxin production is directed at the level of transcription. J Bacteriol 1978;135(2):511-6.
- Ramon G. La floculation dans les mélanges de toxine et de sérum antidiphtérique. Ann Inst Pasteur 1923;37:1001.
- Eaton MD. The Purification and Concentration of Diphtheria Toxin: I. Evaluation of Previous Methods; Description of a New Procedure. J Bacteriol 1936;31(4):347-66.
- Pappenheimer AM. Diphtheria toxin I. Isolation and characterization of a toxic protein from Corynebacterium diphtheriæ filtrates. J Biol Chem 1937;120(2):543-53.
- Kaczorek M, Delpeyroux F, Chenciner N, Streeck RE, Murphy JR, Boquet P, et al. Nucleotide sequence and expression of the diphtheria tox228 gene in Escherichia coli. Science 1983;221(4613):855-8. doi: 10.1126/science.6348945. [Crossref]
- Greenfield L, Bjorn MJ, Horn G, Fong D, Buck GA, Collier RJ, et al. Nucleotide sequence of the structural gene for diphtheria toxin carried by corynebacteriophage beta. Proc Natl Acad Sci U S A 1983;80(22):6853-7. doi: 10.1073/pnas.80.22.6853. [Crossref]
- Choe S, Bennett MJ, Fujii G, Curmi PM, Kantardjieff KA, Collier RJ, et al. The crystal structure of diphtheria toxin. Nature 1992;357(6375):216-22. doi: 10.1038/357216a0. [Crossref]
- Murphy JR, Bishai W, Borowski M, Miyanohara A, Boyd J, Nagle S. Genetic construction, expression, and melanoma-selective cytotoxicity of a diphtheria toxin-related alpha-melanocyte-stimulating hormone fusion protein. Proc Natl Acad Sci U S A 1986;83(21):8258-62. doi: 10.1073/pnas.83.21.8258. [Crossref]
- Falnes PØ, Olsnes S. Cell-mediated reduction and incomplete membrane translocation of diphtheria toxin mutants with internal disulfides in the A fragment. J Biol Chem 1995;270(35):20787-93. doi: 10.1074/jbc.270.35.20787. [Crossref]
- D’Silva PR, Lala AK. Organization of diphtheria toxin in membranes. A hydrophobic photolabeling study. J Biol Chem 2000;275(16):11771-7. doi: 10.1074/jbc.275.16.11771. [Crossref]
- Madshus IH, Wiedlocha A, Sandvig K. Intermediates in translocation of diphtheria toxin across the plasma membrane. J Biol Chem 1994;269(6):4648-52.
- Ren J, Kachel K, Kim H, Malenbaum SE, Collier RJ, London E. Interaction of diphtheria toxin T domain with molten globule-like proteins and its implications for translocation. Science 1999;284(5416):955-7. doi: 10.1126/science.284.5416.955. [Crossref]
- Bennett MJ, Choe S, Eisenberg D. Refined structure of dimeric diphtheria toxin at 2.0 A resolution. Protein Sci 1994;3(9):1444-63. doi: 10.1002/pro.5560030911. [Crossref]
- Louie GV, Yang W, Bowman ME, Choe S. Crystal structure of the complex of diphtheria toxin with an extracellular fragment of its receptor. Mol Cell 1997;1(1):67-78. doi: 10.1016/s1097- 2765(00)80008-8. [Crossref]
- Chenal A, Nizard P, Gillet D. Structure and function of diphtheria toxin: from pathology to engineering. J Toxicol Toxin Rev 2002;21(4):321-59. doi: 10.1081/TXR-120014408. [Crossref]
- O’Keefe DO, Cabiaux V, Choe S, Eisenberg D, Collier RJ. pH-dependent insertion of proteins into membranes: B-chain mutation of diphtheria toxin that inhibits membrane translocation, Glu-349----Lys. Proc Natl Acad Sci U S A 1992;89(13):6202-6. doi: 10.1073/pnas.89.13.6202. [Crossref]
- Audibert F, Jolivet M, Chedid L, Alouf JE, Boquet P, Rivaille P, et al. Active antitoxic immunization by a diphtheria toxin synthetic oligopeptide. Nature 1981;289(5798):593-4. doi: 10.1038/289593a0. [Crossref]
- Boquet P, Alouf JE, Duflot E, Siffert O, Rivaille P. Characteristics of guinea-pig immune sera elicited by a synthetic diphtheria toxin oligopeptide. Mol Immunol 1982;19(12):1541-9. doi: 10.1016/0161-5890(82)90265-6. [Crossref]
- Foss FM. DAB 389 IL-2 (ONTAK): a novel fusion toxin therapy for lymphoma. Clin Lymphoma 2000;1(2):110- 6; discussion 7. doi: 10.3816/clm.2000.n.009. [Crossref]
- Robbins JB, Schneerson R, Trollfors B, Sato H, Sato Y, Rappuoli R, et al. The diphtheria and pertussis components of diphtheria-tetanus toxoids-pertussis vaccine should be genetically inactivated mutant toxins. J Infect Dis 2005;191(1):81-8. doi: 10.1086/426454. [Crossref]
- van der Spoel D, Lindahl E, Hess B, van Buuren A, Apol E, Meulenhoff P, et al. GROMACS user manual version 3.3. 2008. Available from: ftp://ftp.gromacs.org/pub/manual/ manual-3.3.pdf.
- Porollo AA, Adamczak R, Meller J. POLYVIEW: a flexible visualization tool for structural and functional annotations of proteins. Bioinformatics 2004;20(15):2460-2. doi: 10.1093/ bioinformatics/bth248. [Crossref]
- Satpathy R, Guru R, Behera R. Computational QSAR analysis of some physiochemical and topological descriptors of Curcumin derivatives by using different statistical methods. J Chem Pharm Res 2010;2(6):344-50.
- Parrinello M, Rahman A, Vashishta P. Structural transitions in superionic conductors. Phys Rev Lett 1983;50(14):1073-6. doi: 10.1103/PhysRevLett.50.1073. [Crossref]
- Bussi G, Donadio D, Parrinello M. Canonical sampling through velocity rescaling. J Chem Phys 2007;126(1):014101. doi: 10.1063/1.2408420. [Crossref]
- Darden T, York D, Pedersen L. Particle mesh Ewald: An N⋅ log (N) method for Ewald sums in large systems. J Chem Phys 1993;98(12):10089-92. doi: 10.1063/1.464397. [Crossref]
- Hess B, Bekker H, Berendsen HJ, Fraaije JG. LINCS: a linear constraint solver for molecular simulations. J Comput Chem 1997;18(12):1463-72.
- Miyamoto S, Kollman PA. SETTLE: an analytical version of the SHAKE and RATTLE algorithm for rigid water models. J Comput Chem 1992;13(8):952-62. doi: 10.1002/jcc.540130805. [Crossref]
- James JJ, Lakshmi BS, Raviprasad V, Ananth MJ, Kangueane P, Gautam P. Insights from molecular dynamics simulations into pH-dependent enantioselective hydrolysis of ibuprofen esters by Candida rugosa lipase. Protein Eng 2003;16(12):1017-24. doi: 10.1093/protein/gzg135. [Crossref]
- Delmas J, Robin F, Bittar F, Chanal C, Bonnet R. Unexpected enzyme TEM-126: role of mutation Asp179Glu. Antimicrob Agents Chemother 2005;49(10):4280-7. doi: 10.1128/ aac.49.10.4280-4287.2005. [Crossref]
- Kumar A, Purohit R. Computational centrosomics: an approach to understand the dynamic behaviour of centrosome. Gene 2012;511(1):125-6. doi: 10.1016/j.gene.2012.09.040. [Crossref]
- Takeda K, Sasa K, Kawamoto K, Wada A, Aoki K. Secondary structure changes of disulfide bridge-cleaved bovine serum albumin in solutions of urea, guanidine hydrochloride, and sodium dodecyl sulfate. J Colloid Interface Sci 1988;124(1):284-9. doi: 10.1016/0021-9797(88)90349-9. [Crossref]
- Vehlow C, Stehr H, Winkelmann M, Duarte JM, Petzold L, Dinse J, et al. CMView: interactive contact map visualization and analysis. Bioinformatics 2011;27(11):1573-4. doi: 10.1093/bioinformatics/btr163. [Crossref]
- Bozorgmehr MR, Housaindokht MR. Effects of sodium dodecyl sulfate concentration on the structure of bovine carbonic anhydrase: molecular dynamics simulation approach. Rom J Biochem 2010;47:3-15.
- Housaindokht MR, Monhemi H. The open lid conformation of the lipase is explored in the compressed gas: new insights from molecular dynamic simulation. J Mol Catal B Enzym 2013;87:135-8. doi: 10.1016/j.molcatb.2012.11.003. [Crossref]
- Galzitskaya OV, Reifsnyder DC, Bogatyreva NS, Ivankov DN, Garbuzynskiy SO. More compact protein globules exhibit slower folding rates. Proteins 2008;70(2):329-32. doi: 10.1002/prot.21619. [Crossref]
- Tsai CJ, Lin SL, Wolfson HJ, Nussinov R. Studies of protein-protein interfaces: a statistical analysis of the hydrophobic effect. Protein Sci 1997;6(1):53-64. doi: 10.1002/pro.5560060106. [Crossref]
- Tsai CJ, Nussinov R. Hydrophobic folding units derived from dissimilar monomer structures and their interactions. Protein Sci 1997;6(1):24-42. doi: 10.1002/pro.5560060104. [Crossref]
- Makarov DE, Keller CA, Plaxco KW, Metiu H. How the folding rate constant of simple, single-domain proteins depends on the number of native contacts. Proc Natl Acad Sci U S A 2002;99(6):3535-9. doi: 10.1073/pnas.052713599. [Crossref]